Enterprises attempting to leverage Artificial Intelligence (AI) and Machine Learning (ML) technologies face significant hurdles. Surveys indicate that almost 60% to 85% of AI/ML models never reach deployment. The root causes include the need for multidisciplinary collaboration, data quality improvements, and preparation for post-production needs and challenges.
AI Assembly
Framework for Successful AI/ML Adoption
Overview
Challenge
60% to 80%
Challenges for AI/ML Adoption
Clear Business Objectives & Strategy
Many enterprises pursue AI/ML initiatives based on a broad understanding of potential use cases, lacking specificity, measurability, and viability.
Data Quality and Management
Ensuring the availability, accuracy, and completeness of data is critical, yet often difficult to achieve
Multidisciplinary Collaboration
Successful AI/ML projects require close collaboration between data scientists, engineers, domain experts, and business stakeholders, which can be challenging to coordinate
Scalability
Scaling AI/ML models from pilot projects to full production can be technically challenging and resource-intensive
Monitoring and Maintenance
Post-deployment, AI/ML models need continuous monitoring, maintenance, and updates to remain effective and accurate
Talent and Skill Gaps
There is a high demand for skilled AI/ML professionals, making it difficult to find and retain the necessary talent
AI Assembly Framework
AI Assembly, an enterprise AI/ML adoption framework, addresses these obstacles and streamlines the path to AI/ML adoption.
Its structured methodology focuses on collaboration, innovation, and practical implementation, guiding enterprises from initial exploration to successful operational integration.
This framework brings together business, data, and IT teams with data scientists and engineers. Together, they invest time and resources to identify, prioritize, and develop use cases based on their impact, viability, and data availability. Once identified, the framework provides a well-defined, iterative approach to deliver AI/ML-powered outcomes to production.
Three Phases of AI Assembly
Invest Phase
- Testing
- Validation of AI/ML projects
- MLOps strategy formulation
- Explainability
- Risk assessment
Develop Phase
- Cleansing
- Analysis
- Feature engineering
- Model training
- Deployment
Harvest Phase
- Governance
- Training
- Communication
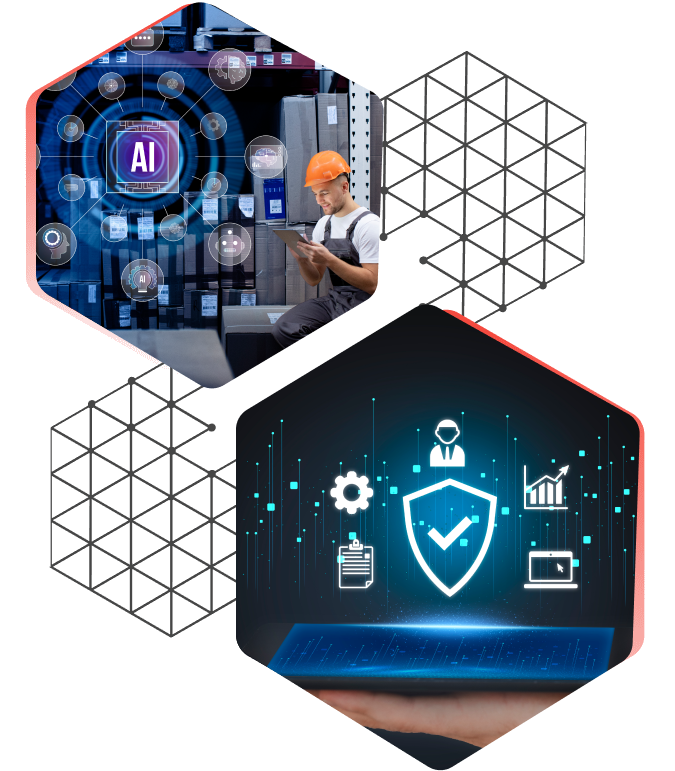
Key Advantages of AI Assembly
- Accelerated Time to Market: Speed up from several months to weeks
- Lower Risk of Failure: Test and validate use cases with stakeholders
- Cost Savings: Smart planning and resource efficiency for maximum ROI
- Trust and adoption: Comprehensive data management for accuracy
- Competitive Advantage: Continuous Optimization for dynamic business needs
Download the complete the AI Assembly Guide
Why GS Lab | GAVS as Implementation Partner
Multidisciplinary teams
We have a cohesive interdisciplinary team experienced with multiple end-to-end AI/ML projects
Pragmatic Approach
We help establish feasibility with rudimentary models and then implement a complete pipeline till model deployment and monitoring
Domain led Solutions
Our deep domain understanding across healthcare, supply chain, Life Sciences, pharm, hi-tech and BFSI helps us carve industry-centric solutions for our customers
Higher Success Rate
With an 85% success rate for AI/ML projects, we ensure your investments meet your end goals
Modular Services
Solutions and services carved specifically for each stage of AI Assembly
Innovative Business Models
Equally invested in partnership with shared risk