“It is the best of times, it is the worst of times; everything is automated, everything is complicated; it is the age of human limitlessness, it is the age of artificial intelligence; it is the epoch of real-time data, it is the epoch of generated data” – adapting Dickens to the fictitious reality of our times!
The increasing adoption of digital therapeutics by the pharmaceutical industry is leading to significant innovations that are enhancing patient outcomes. However, investments in this space are a result of the fortitude of a few forward-thinking innovators and early adopters. It is a huge conceptual challenge to align digital therapeutics to prescription therapeutics since such initiatives require a comprehensive and multi-faceted approach involving credibility building, value demonstration, and stakeholder education.
Between now and the end of this decade, two events in tandem will push the spend on digital therapeutics from discretionary to essential.
The first revolves around several blockbuster drugs losing their protective patents and exclusivity during this period. This shift will compel pharmaceutical companies to prioritize product differentiation for competitive advantage and look beyond drug efficacy and effectiveness. Consequently, the industry will increasingly embrace a digital and patient-centric approach, focusing on patient engagement and empowerment, personalized care plans, tailored interventions, evidence-based practices, and real-time data for insights and treatment support. Collaborations with healthcare providers and strategic plans for reach and access to care institutions, healthcare professionals, and patients will be prevalent.
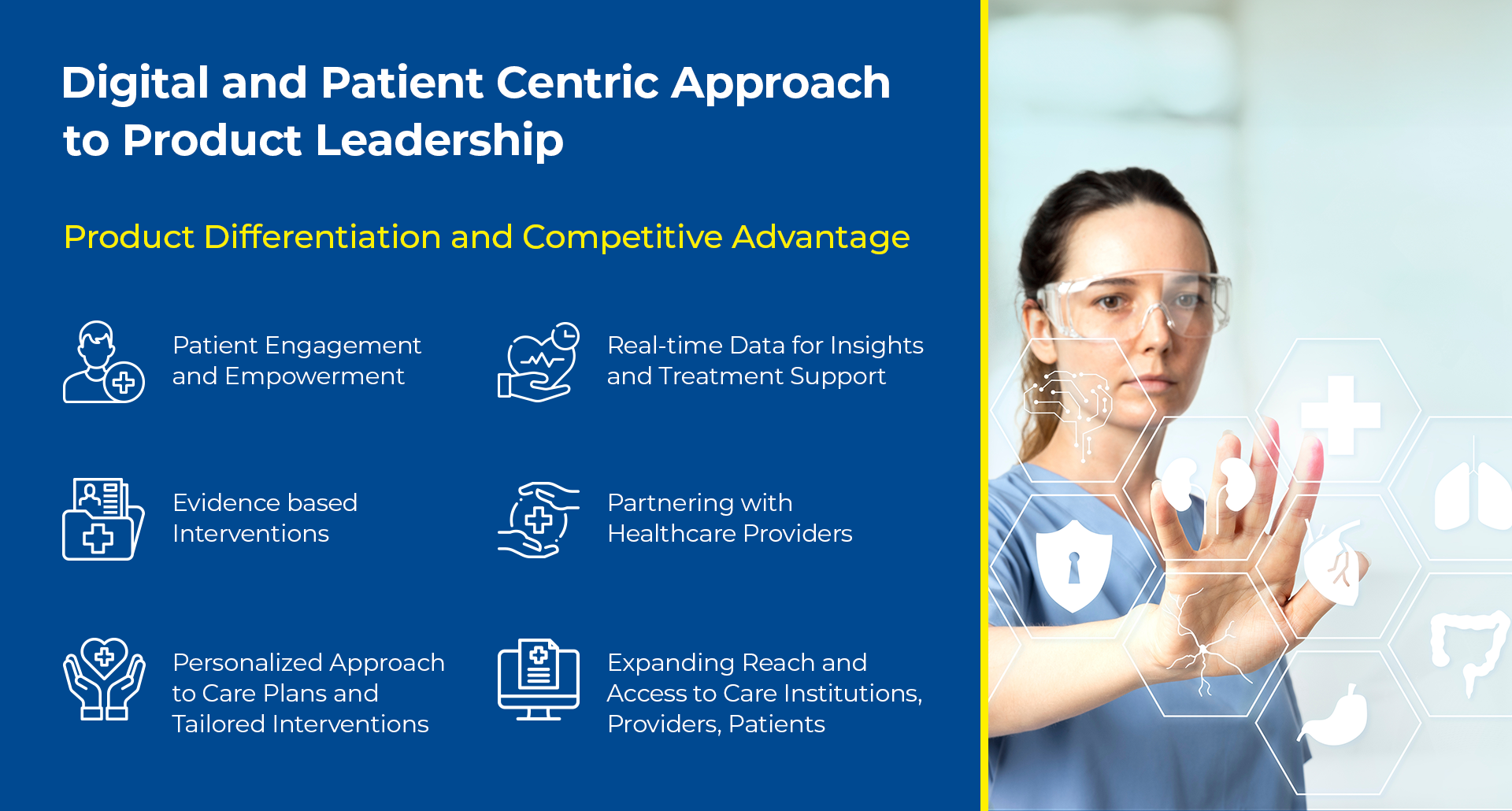
The second is the rapid evolution of Artificial Intelligence (AI) and Machine Learning (ML) technologies, now unavoidable, with far-reaching disruptive potential across industries and business functions. In the context of digital therapeutics, AI and ML bring about game-changing advancements. Digital therapeutics, viewed as digital applications that complement and differentiate treatment pathways and drug offerings, will serve as catalysts for patient engagement and physician enablement. AI and ML-driven digital therapeutics will have the capability to not only track but also predict clinical outcomes, proactively make evidence-based interventions, and extend remote patient monitoring beyond conventional parameters.
Digital Therapeutics (DTx) to AI Driven Digital Therapeutics (DTx.AI)
Standard DTx architectures focused on capturing and analyzing real-world patient data to support personalized care through mobile and web platforms. They enabled real-time data collection from wearables, devices, sensors, and medical equipment while facilitating connectivity with Electronic Medical Records (EMRs). They also offered data analytics, insights, treatment evaluation, monitoring tools, and extended support for cognitive behavioral therapy, gamification, lifestyle management, and wellness awareness.
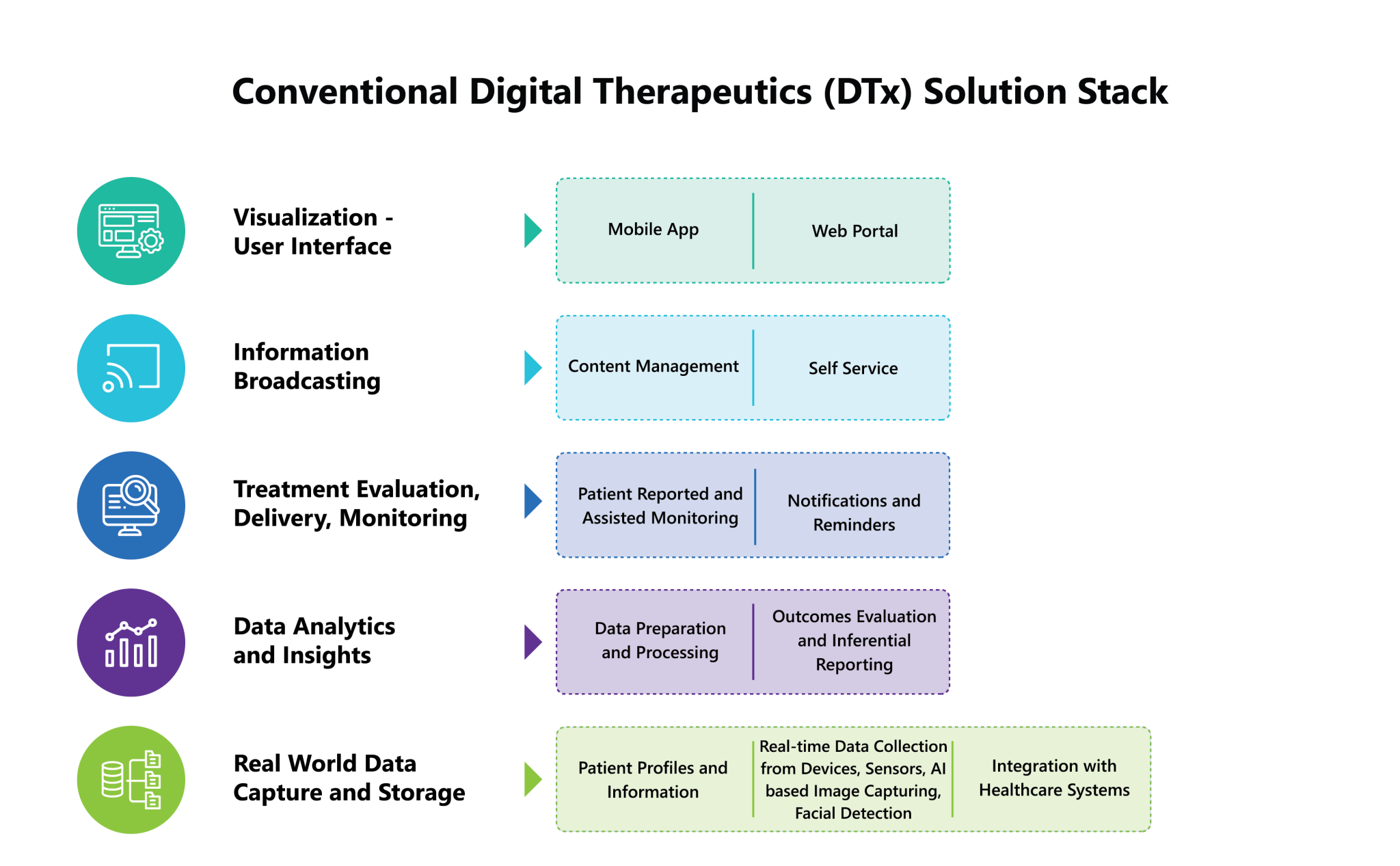
DTx.AI incorporates therapeutic algorithms and machine learning models for personalized algorithmic decision making based on evidence-based validation. Machine learning techniques enable faster and more precise learning from data sets, identification of patterns, and correlations that recommend personalized treatment plans based on individual patient characteristics. AI algorithms can analyze various patient data, including medical history, genetic information, and lifestyle factors. By continuously monitoring and adjusting treatment plans, AI-powered digital therapeutics optimize treatment efficacy while minimizing adverse effects.
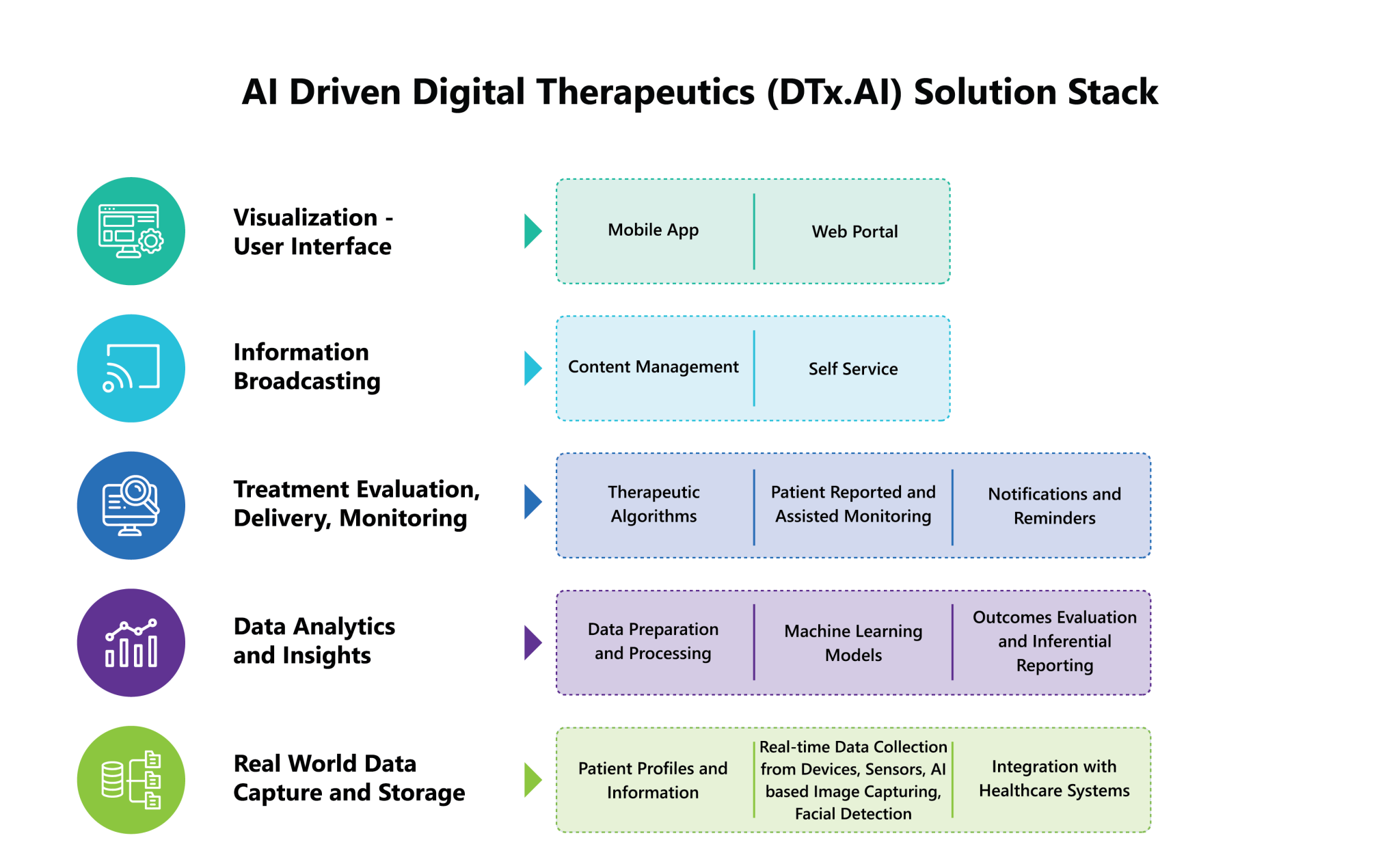
Initiatives leveraging AI in digital therapeutics help detect potential adverse events and drug interactions and contribute to ongoing pharmacovigilance and drug safety monitoring. AI enhances the reliability of remote patient monitoring and disease management by swiftly and accurately identifying patterns, deviations, and anomalies. Through the integration of appropriate alert systems, AI ensures timely notifications and alerts.
Furthermore, AI transforms DTx from being lifestyle and behavioral assistance tools to becoming active unassisted behavior modification and cognitive therapy support systems for patients. It enables information-driven and interactive engagements, offering comprehensive and personalized experiences.
Digital Therapeutics and Generative AI (DTx.GenAI)
The adoption of DTx.AI is paving the way for readiness and integration with generative AI. Although ethical, regulatory, reliability, and bias concerns require careful navigation, generative AI introduces the next level of possibilities.
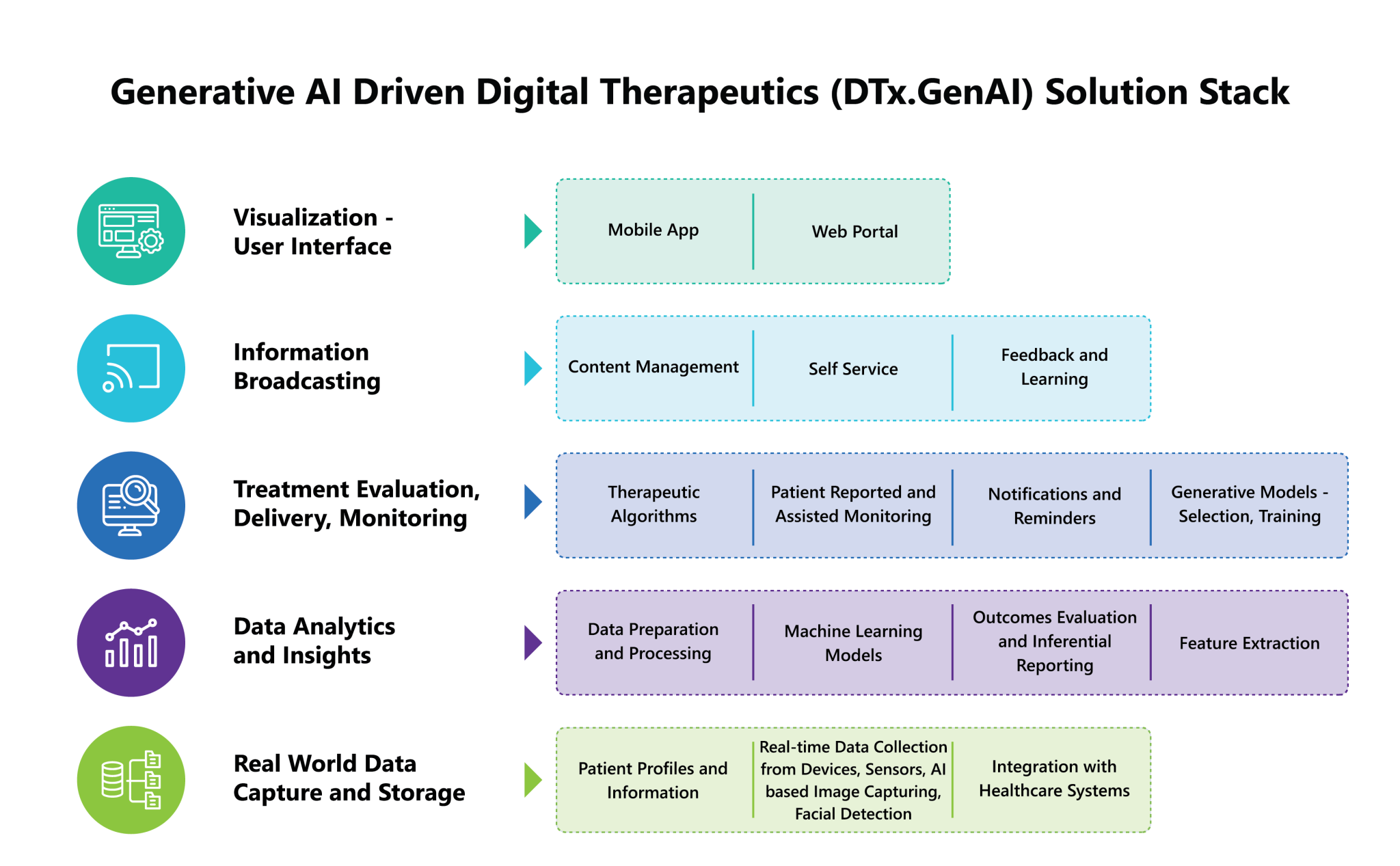
Generative AI in Digital Therapeutic solutions (DTx.GenAI) enhances and refines machine learning models through feature extraction capabilities. By incorporating Natural Language Processing (NLP) and image processing, DTx.GenAI identifies and extracts only the necessary data sets and patterns for functioning of the models. This continuous learning, fine tuning, selection, and extraction process helps imbibe new data quickly to bring in dynamic improvements in therapeutic interventions.
DTx.GenAI will leverage generative models for selection and training, utilizing deep learning, reinforcement learning, and autoencoders for model selection. These capabilities facilitate the creation of new content resembling real-world data/ content from the machine learning model, and existing data. The training capability helps create newer data/ content resembling real world data/ content. A constant quest between creating close-to-real data and distinguishing real from created data helps refine the model. This generative AI capability enables simulations and training for patients and healthcare providers, optimizing treatment plans and post-treatment care. For pharmaceutical companies, this capability helps arrive at insights and predictive analytics, extrapolating beyond the available real population and data.
With DT.GenAI focusing on generating data rather than simply making decisions or predictions, the future of digital therapeutic solutions will heavily depend on user interactions and inputs. Feedback mechanisms and surveys will play a vital role in analyzing effectiveness and fine-tuning the models.
The ultimate payback is the possibility to extend beyond the treatment under focus. Comprehensive analysis encompassing commercial launches, real-world data, past trials data, genomic and proteomic information, uncovers more precise correlations, provides insights for target identification and refinement of molecules in drug discovery, and influences trial protocol designs for new treatment options or other therapeutic areas.
A Customer Success Story
This case study highlights our digital therapeutics intervention for a customer who has transformed biochemical investigations for oncology patients, enabling them to perform these tests from the comfort of their own homes.
Overview
Patients undergoing chemotherapy require regular urine tests, which traditionally involves visiting an out-patient facility or lab for analysis. Our customer’s goal was to minimize delays and enhance convenience for these patients by enabling them to complete the entire test remotely.
The Solution
The team of expert data scientists and AI/ML engineers from GS Lab | GAVS enabled the customer to successfully revolutionize remote patient monitoring for oncology patients post chemotherapy through an ML enabled remote urine analysis system. The solution delivers convenience, speed, and efficiency to traditional laboratory tests.
To turn the customer’s vision into reality, GS Lab | GAVS had to overcome several challenges. It was important to create an intuitive and seamless user experience to cater to non-tech-savvy users and patients who are quite often under a lot of emotional stress after chemotherapy. The solution had to be compatible with a wide variety of mobile devices and laptops for better accessibility. Concerns relating to data access controls, confidentiality, secure data transmission and storage had to be adequately addressed. The accuracy of the ML model needed to match the level of credibility expected by medical experts. Extracting relevant features from urine test strip images required deep image processing knowledge, considering factors like noise and variability.
ML and Data Science Process
To develop this ML-based remote urine analysis solution, a systematic data science process was adopted that involved choosing the right ML model after experimentation, extensive sample data collection to train the model, development of applications to capture the test strip images, extraction of relevant features from the images, handling of noise and variability, real-time transmission of results, and user-friendly, diagnostic reporting. Anonymized data was stored and made available for further research. Effective data engineering ensured seamless flow of data throughout the system encased within strict regulatory compliance, data protection, and documentation.
Machine learning and advanced technologies helped provide instant diagnostic reports, facilitated communication with doctors, and streamlined payment processes. Additionally, the data collected across the patient population was used for analytics while ensuring patient confidentiality.
You can find more details on the solution here
https://www.gslab.com/downloads/Remote-urine-analysis-for-cancer-patients-at-home-using-machine-learning.pdf
To find out how the solution can be customized for your specific needs, please reach out to marketing@gslab.com
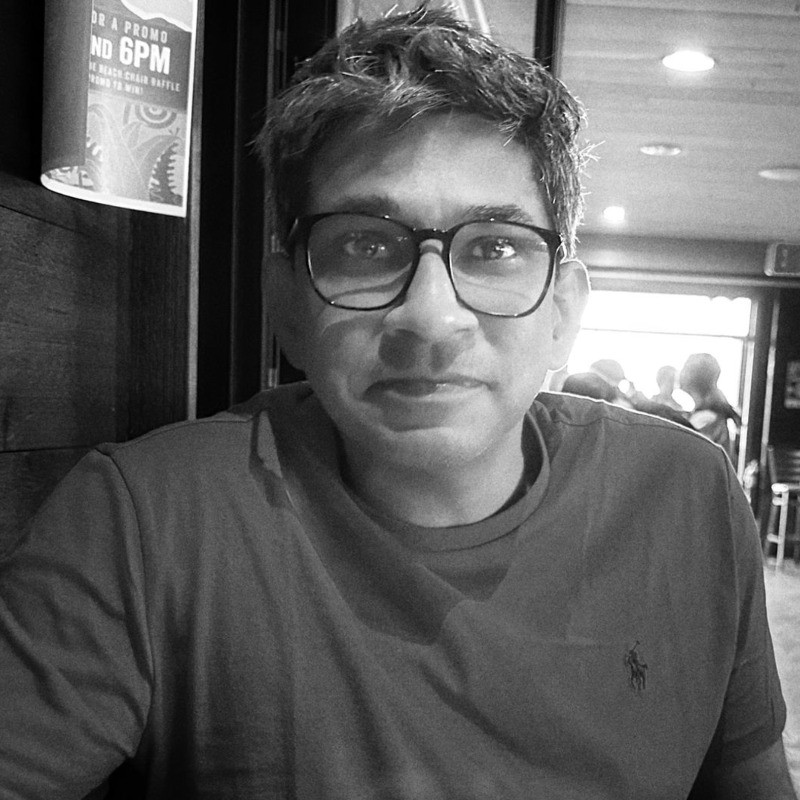
Author
Dr. Vinod Sanjay
Dr. Vinod Sanjay leads the Life Sciences practice at GS Lab | GAVS. His focus is on shaping digital ecosystems and next-generation business models for Life Sciences. As a consulting and technology partner, he has led several high-impact consulting engagements and technology transformation projects.