What’s the common thread between delivering the highest possible customer value (external-facing) and achieving operational efficiency (inward-facing)? What underlies both of these key areas is ‘useful analytics’. Companies need to have their hand-on-the-pulse and take corrective actions to stay on course. Data is one of the most important levers to make it happen. But it’s not just any or all data coming in – the data needs to be contextualized to be actionable. How does one go from raw data to discovering insights and applying them?
A framework to better understand this journey can be broken down into four key steps:
Monitoring: “What is happening?” This is where data coming in from various sources is ingested, processed, and displayed in intuitive ways for humans to consume. The data sources are numerous, and of a wide variety – systems of record (ex: sensors, user profile data, manual inputs) and systems of interaction (ex: transactions, processes, test data). The data formats are wide-ranging too – numerical data, text, images, video, voice, etc. In a hospital, this could be the current occupancy in the Intensive Care Unit or the number of patients and their wait times in the ER.
The key to getting monitoring right is to focus on the Velocity and Veracity of the data. Is it reliable, and is it becoming available in near-real-time? The value of monitoring goes down if either of those is missed. Other aspects which need to be managed are the data volume, formats, metadata for further analytics, etc.
Diagnostics: “Why is it happening?” A well-designed monitoring system highlights what is going well, and especially what is starting to go off-track. It helps zoom in on the process variations, the anomalies, and this is where Diagnostics can help answer the “Why” behind the system behavior. The tools should be able to help an expert look through past data, visualize trends, and juxtapose various relevant parameters to uncover the domain-specific reasons behind the anomalies.
To get diagnostics right, the system should allow the domain expert to quickly navigate these aspects, so that they can contextualize the observation. Finding the right correlations (or simply, parameters which are moving together) then helps diagnose the issues.
In a hospital, process diagnostics will help figure out why there is a sudden uptick in ER wait times.
Prediction: “What is likely to happen next?” Once we have a handle on what’s happening and why at times the process falters, we can get better at predicting component and system behavior. Predictive machine learning models can be developed to make this process automated and smarter.
Prediction is not just about extending the trend line. It’s about the expert’s and the model’s ability to consider causes of variation, the interconnectedness of parameters and user behavior, sources of breakdowns, and leave room for the ever-present fluctuations. The predictive algorithms should be able to correlate parameter change before a breakdown and flag a potential snag, thereby alerting the operators beforehand. This is where a lot of value is created since the prevention of a breakdown reduces downtime and allows proactive fixing of the underlying problem.
For example, in a hospital scenario, this could be predicting patient readmission, or for a pharmacy – predicting demand changes for certain critical drugs seasonally.
Prescription: “Change this to make the right thing happen.” The final step is tying all the insights gathered till now, and advising on the next steps. The predictive models have to work as the domain expert’s assistants and give recommendations for smoother operations. This is the hardest but also likely the most valuable piece. It improves the productivity of the human operators, and truly leverages the power of technology to improve the outcomes. It is not about replacing a human expert, but complementing their skills to cut down the time and effort spent on each individual problem.
Hospital staff can be helped by suggesting changes in patient flow, highlighting bottlenecks to resolve, or getting to the root cause of patient readmissions for a specific type of disease and patient demographic.
Following this framework helps us move from mere reporting of the data to leveraging it to gain business benefits. If the insights are not being discovered and not being put in context to make them actionable, there is no benefit derived from the entire analytics exercise. Moreover, it helps to move focus away from the specific tools (the “plumbing”) to the value chain (the “water flow”) – where value is created and how it’s transferred to the end users.
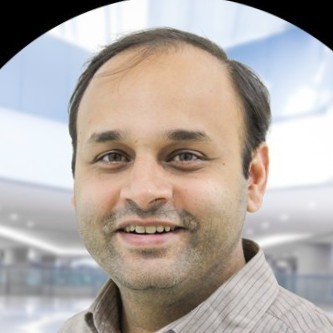
Author
Mandar Gadre